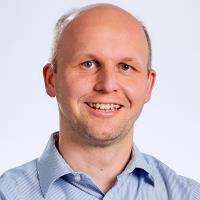
The potential of AI/ML is immense, yet it is often misused by managers who are driven by trends and a fear of missing out. Like any technology, AI/ML should be seen as a means to an end and should be evaluated for its business value within the appropriate context.
To make informed decisions, managers should begin by assessing the use case they want to address and evaluating the associated business value. This evaluation should be conducted independently of considering the use of AI/ML. Unfortunately, the decision to use AI/ML is often the first one made.
When determining the suitability of AI/ML, it is essential to have a clear understanding of its definition. AI/ML relies on complex models with thousands or even millions of parameters, fine-tuned through training. It excels in situations where:
-
There are large datasets available for training the model (or your use case fits an already trained model offered by a third-party company).
-
It is challenging to define rules that can convert input parameters to desired results. For instance, converting spoken language to text cannot be easily achieved through a small set of rules.
These critical conditions are often overlooked:
-
Models trained on insufficient or unrepresentative datasets will yield poor results.
-
If a limited set of rules (forming an algorithm) can achieve the desired outcome, it is often more effective to code those rules instead of using AI/ML. Rule-based systems are easier to interpret, maintain (fixing an issue can be done by adapting a rule, while an AI/ML model will need to be retrained, potentially leading to unexpected regressions on other cases), and are less computationally intensive. There is a tipping point where the number of rules becomes too extensive, making it more practical to employ an AI/ML model. Additionally, AI/ML models may identify correlations and rules that were not initially considered, leading to better results. However, comparing the cost, maintenance, and outcome quality between AI/ML models and traditional rule-based systems is challenging. Conducting extensive and expensive A/B tests with both models over a significant period is usually not feasible for organizations.
For instance, in the case of a bank wanting to establish a new credit-scoring model, a rule-based scoring algorithm can be implemented based on predefined factors such as Loan to Value (LTV), Debt to Income (DTI), and risk and stability factors like customer loyalty, customer age, and residential history. Alternatively, an AI/ML model could incorporate a broader range of input factors, e.g. full account transaction history and social media details. The AI/ML model may provide better results, especially for individuals with unconventional profiles. However, it also introduces challenges such as complex data pipelines, data cleaning, and difficulties in explaining or defending specific credit decisions (with high risk of discrimination bias).
Measuring the performance of this credit-scoring AI/ML model versus the traditional rule-based model is very challenging:
-
The impact of credit decisions takes years to unfold, as the “credit default rate” will be impacted by credit decisions of several years before.
-
Only the approved credits can be compared; if a model refuses a credit, you can never know if this credit would not have been reimbursed without any issues.
Another good example is the use of AI/ML for Recommendation engines. In many cases, a simple database query based on user similarities (e.g. people buying similar product or people with same age, family situation, profession, hobbies/interests…​.) or product associations (products bought together) can yield comparable results to complex AI/ML models.
In general, AI/ML excels in handling unstructured data, like speech records, documents, unstructured text, images, where traditional software development struggles. However, these AI/ML use cases also pose higher complexity due to extensive data preparation, complex models, and challenges in interpretation and verification. Consequently, many companies focus on AI/ML models that utilize structured data. These models rely on statistical correlations and may not always provide obvious or substantial added value compared to traditional rule-based systems. The hype around AI/ML can sometimes lead to these use cases being promoted, although the benefits may be non-existent or very poor.
To maximize the value of AI/ML, organizations should carefully assess where to deploy AI/ML and where not. Organisations should avoid going for quick AI/ML implementations, which can just as well be implemented by traditional software development. Instead they should assess more complex use cases, where the true potential of AI/ML can make a significant difference. Often those more interesting use cases can however be handled quite well by third-party pre-trained models (often offered as easy to integrate APIs), meaning for many companies there is no immediate need to hire AI/ML specialists. Instead it might be more interesting to train everyone within the organisation in the possibilities and constraints of AI/ML and have a good knowledge of which third-party AI/ML services are available on the market.
- SEO Powered Content & PR Distribution. Get Amplified Today.
- PlatoData.Network Vertical Generative Ai. Empower Yourself. Access Here.
- PlatoAiStream. Web3 Intelligence. Knowledge Amplified. Access Here.
- PlatoESG. Carbon, CleanTech, Energy, Environment, Solar, Waste Management. Access Here.
- PlatoHealth. Biotech and Clinical Trials Intelligence. Access Here.
- Source: https://www.finextra.com/blogposting/25029/the-right-fit-assessing-business-value-before-adopting-aiml?utm_medium=rssfinextra&utm_source=finextrablogs